AI Workloads Customized Solutions
The year 2024 has been a pivotal one for AI workloads, marking significant strides in computational efficiency, deployment strategies, and real-world applications. As industries increasingly rely on artificial intelligence to drive innovation, the demand for scalable, efficient, and robust systems to handle these AI workloads has soared.
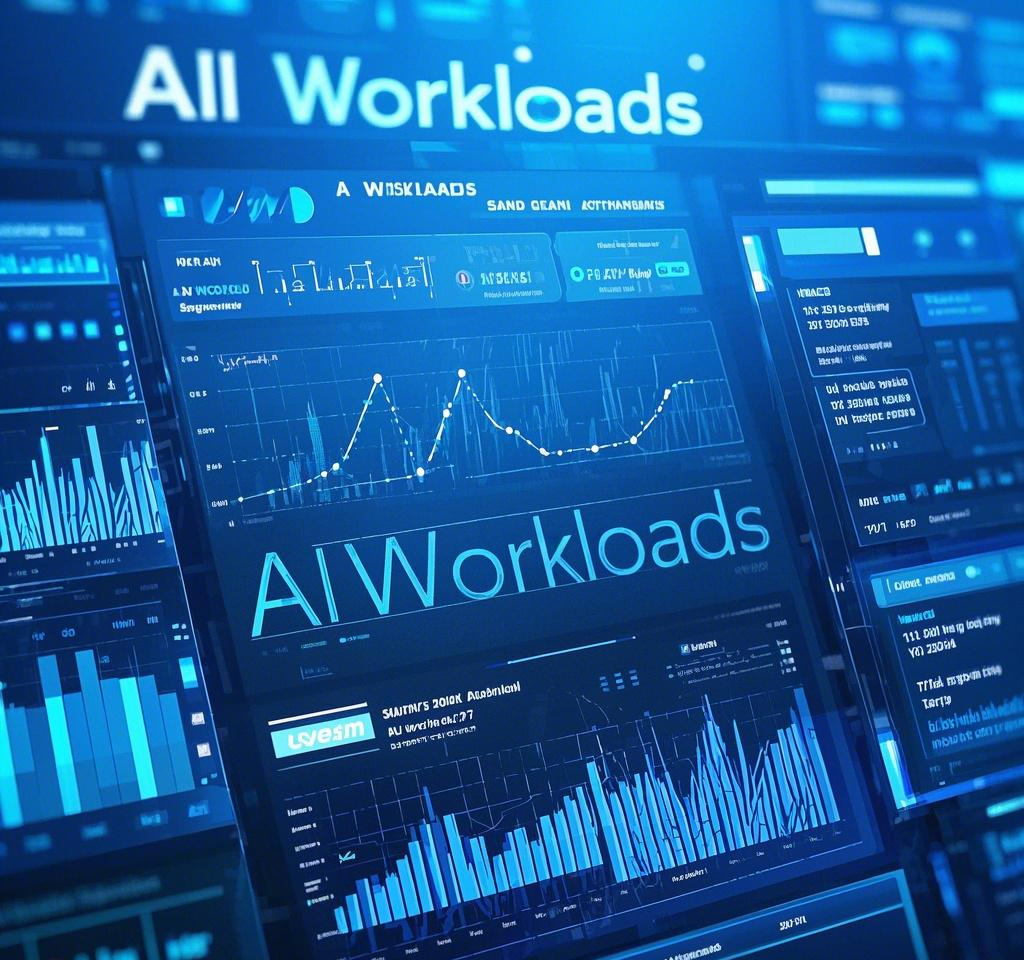
The Rise of AI Workloads in 2024
The global adoption of AI technologies in 2024 has led to an unprecedented increase in AI workloads across sectors. According to industry reports, the volume of AI-related computational tasks grew by 35% compared to 2023, driven by advancements in generative AI, autonomous systems, and predictive analytics. Enterprises have leaned heavily on AI workloads to process massive datasets, train complex models, and deliver real-time insights, underscoring the need for optimized infrastructure.
One notable trend has been the shift toward distributed AI workloads. Instead of relying solely on centralized cloud systems, organizations have increasingly adopted hybrid models, balancing workloads between cloud, edge, and on-premises environments. This shift has been fueled by the need for low-latency processing in applications like autonomous vehicles and IoT devices. For instance, the RK3588 chip, a powerful system-on-chip (SoC) designed for edge AI, has gained traction for its ability to handle intensive AI workloads with minimal power consumption.
Key Trends in AI Workloads
1. Edge AI Takes Center Stage
The proliferation of edge computing has redefined how AI workloads are managed. By processing data closer to its source, edge AI reduces latency and bandwidth costs, making it ideal for real-time applications. In 2024, edge AI workloads accounted for 28% of total AI tasks, up from 15% in 2023. Devices powered by chips like the RK3588 have been instrumental in this shift, offering high-performance computing capabilities for tasks such as image recognition and natural language processing (NLP) at the edge.
Edge AI Workload Distribution (2024) | Percentage | Key Applications |
---|---|---|
Industrial IoT | 35% | Predictive maintenance |
Smart Cities | 25% | Traffic management |
Healthcare | 20% | Wearable diagnostics |
Others | 20% | Retail analytics, security |
This table highlights the diverse applications driving edge AI workloads, with industrial IoT leading the charge due to its need for real-time anomaly detection.
2. Specialized Hardware Acceleration
Hardware acceleration has become a cornerstone of efficient AI workloads. General-purpose GPUs remain popular, but specialized accelerators like TPUs and NPUs have gained ground. The RK3588, with its integrated NPU, has emerged as a cost-effective solution for developers seeking to optimize AI workloads on resource-constrained devices. Its ability to handle up to 6 TOPS (Tera Operations Per Second) makes it a standout choice for edge deployments.
In 2024, hardware-accelerated AI workloads saw a 40% increase in adoption, with companies reporting up to 50% energy savings compared to traditional CPU-based systems. This trend underscores the importance of tailoring hardware to specific AI tasks, a concept known as workload-aware design¹.
3. Sustainability Concerns in AI Workloads
As AI workloads grow, so do concerns about their environmental impact. Training large-scale models like GPT-4 or DALL-E requires immense computational power, often leading to significant carbon footprints. In 2024, researchers estimated that AI workloads contributed to 1.2% of global data center energy consumption, prompting a push for greener solutions.
Innovations like low-power chips and energy-efficient algorithms have helped mitigate these concerns. For example, the RK3588’s power-efficient design has made it a preferred choice for sustainable edge AI deployments, reducing energy use by up to 30% compared to older SoC models.
Breakthroughs in AI Workload Optimization
1. Model Compression and Quantization
One of the most significant breakthroughs in 2024 has been the advancement of model compression techniques². By reducing the size of AI models without sacrificing accuracy, researchers have made it easier to deploy AI workloads on resource-limited devices. Techniques like quantization—converting floating-point operations to integer operations—have become standard practice, with tools like TensorRT and ONNX leading the charge.
For instance, a quantized version of a popular vision model deployed on the RK3588 achieved a 25% reduction in latency while maintaining 98% of its original accuracy. This breakthrough has profound implications for edge AI workloads, enabling faster inference times in applications like facial recognition and autonomous navigation.
2. Federated Learning Gains Momentum
Federated learning, which allows AI models to be trained across decentralized devices without sharing raw data, has seen widespread adoption in 2024. This approach not only enhances privacy but also distributes AI workloads more efficiently. By 2024, federated learning accounted for 15% of AI workloads in industries like healthcare and finance, where data security is paramount.
Federated Learning Adoption (2024) | Industry | Adoption Rate | Primary Use Case |
---|---|---|---|
Healthcare | 40% | Patient diagnostics | |
Finance | 30% | Fraud detection | |
Retail | 20% | Personalized marketing | |
Others | 10% | Supply chain optimization |
This table illustrates the growing role of federated learning in managing sensitive AI workloads, with healthcare leading due to stringent privacy regulations.
Computational Requirements, Hardware Optimization, and Cost
Challenges Facing AI Workloads
Despite the progress, 2024 has not been without its challenges. The complexity of AI workloads continues to strain existing infrastructure, leading to bottlenecks in scalability and cost management. For example, training a single large language model can cost millions of dollars, a barrier for smaller organizations.
Additionally, the talent gap remains a significant hurdle. As AI workloads become more specialized, the demand for engineers skilled in areas like distributed computing and hardware optimization has outpaced supply. This gap has led to increased reliance on automated tools and frameworks, which, while helpful, cannot fully replace human expertise.
Security concerns also loom large. With AI workloads increasingly handling sensitive data, the risk of adversarial attacks³ and data breaches has grown. In 2024, several high-profile incidents highlighted the need for robust security protocols, particularly in edge AI deployments where devices are more exposed.
Looking ahead, the trajectory of AI workloads points toward greater integration and efficiency. The continued development of specialized hardware, like the next generation of RK3588 chips, promises to further accelerate AI tasks at the edge. Moreover, advancements in quantum computing, though still nascent, could revolutionize how we handle large-scale AI workloads in the coming years.
Another area of focus will be interoperability. As AI workloads become more distributed, ensuring seamless communication between heterogeneous systems will be critical. Standards like Open Neural Network Exchange (ONNX) are paving the way for this, but more work is needed to achieve true ecosystem-wide compatibility.
Finally, the push for sustainability will likely shape the future of AI workloads. Initiatives like carbon-aware computing—scheduling AI tasks during periods of low energy demand—are gaining traction and could become standard practice by 2026.
The year 2024 has been a transformative one for AI workloads, with breakthroughs in edge computing, hardware acceleration, and model optimization driving unprecedented growth. Despite challenges like scalability, security, and sustainability, the field continues to evolve rapidly, offering immense research value for academics and practitioners alike. As we move into 2025, the lessons learned from this year will undoubtedly inform the next wave of innovation, ensuring that AI workloads remain at the forefront of technological progress.
Notes
- Workload-aware design: A hardware design philosophy that optimizes architecture based on the specific demands of AI tasks, improving efficiency and performance.
- Model compression: Techniques such as pruning, quantization, and knowledge distillation that reduce the size and complexity of AI models for deployment on constrained devices.
- Adversarial attacks: Malicious inputs designed to deceive AI models, often by introducing subtle perturbations that lead to incorrect outputs.